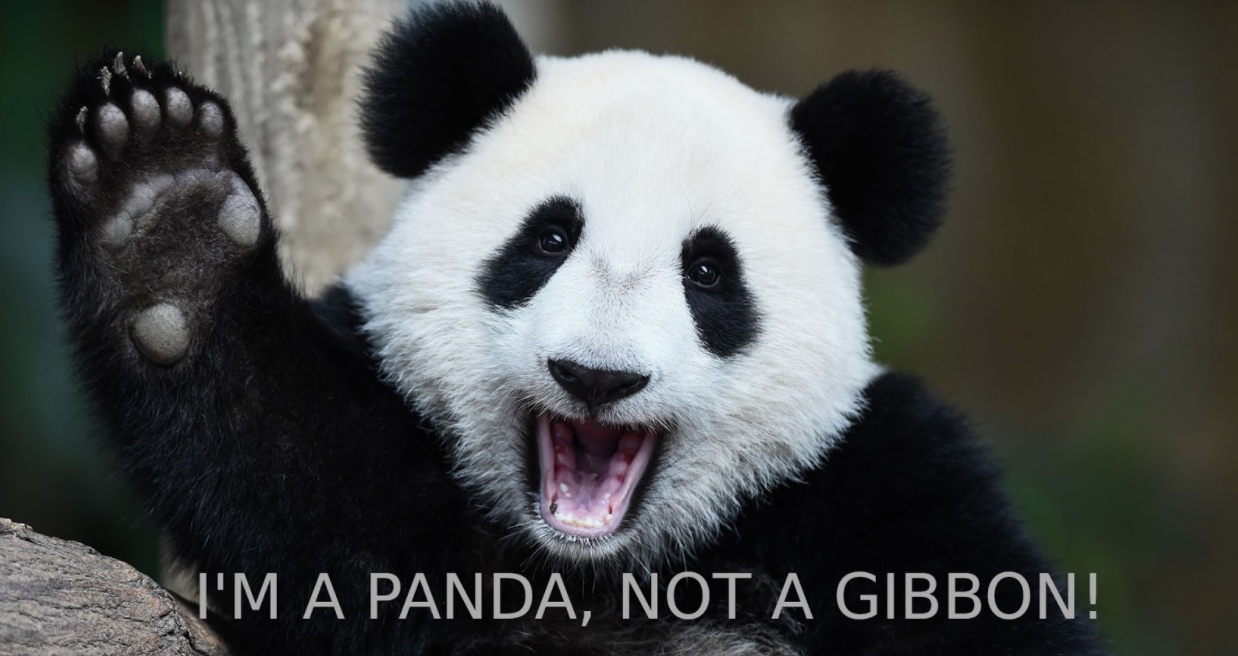
Secure sensing in AIoT
Main contributors from the group: Qun Song (topic coordinator), Zhenyu Yan
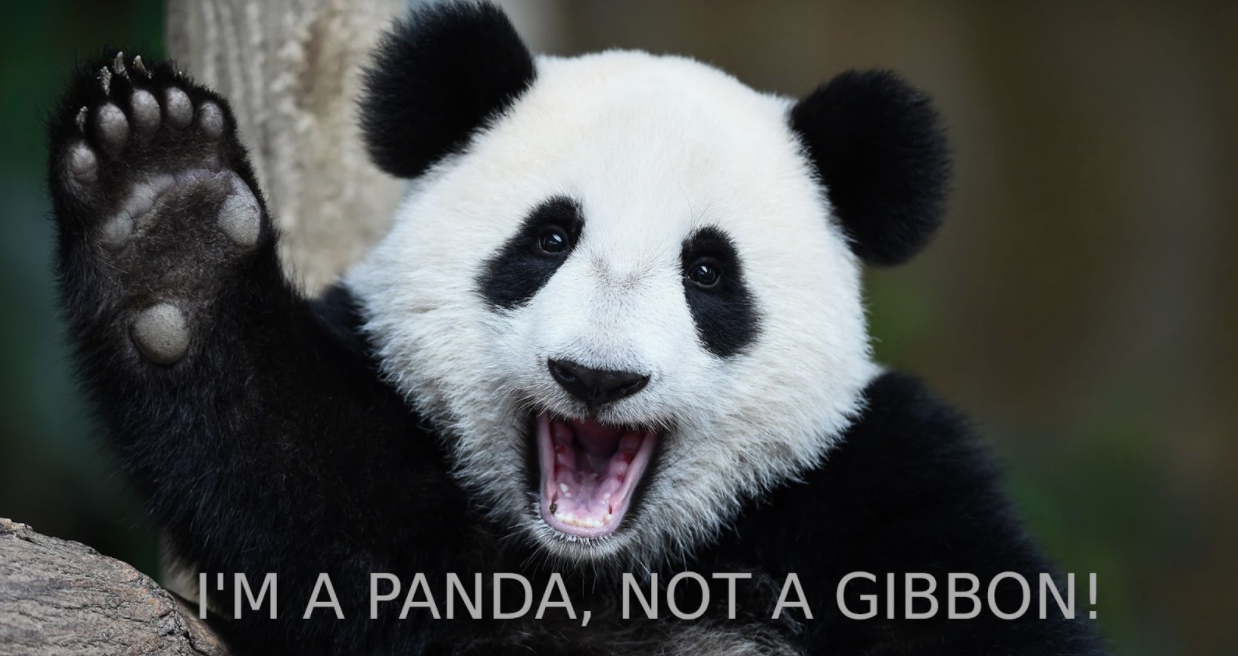
Image modified from this, credit goes to the source. Also refer to this paper for background
By 2025, it is estimated that there will be more than 41.6 billion networked IoT devices. These IoT devices generate 79.4 zettabyes data yearly, which is almost twice of today’s whole Internet (44 zettabytes). Transmitting such massive IoT data to the clouds for centralized processing will face communication bandwidth bottleneck. In addition, the communication networks will introduce uncertain time delays that are undesirable for many applications. To cope with these grand challenges, edge computing moves the computation on the IoT data closer to the data sources. Specifically, the IoT sensors and the last-mile data aggregators, acting as edge nodes, will undertake a significant portion of the computation on the IoT data; only data summaries and commands will be exchanged between the edge nodes and the cloud servers through the Internet when needed. As such, a hybrid computing paradigm consisting of edge computing at the front end and cloud computing at the back end will prevail along with the formation of IoT as a global infrastructure.
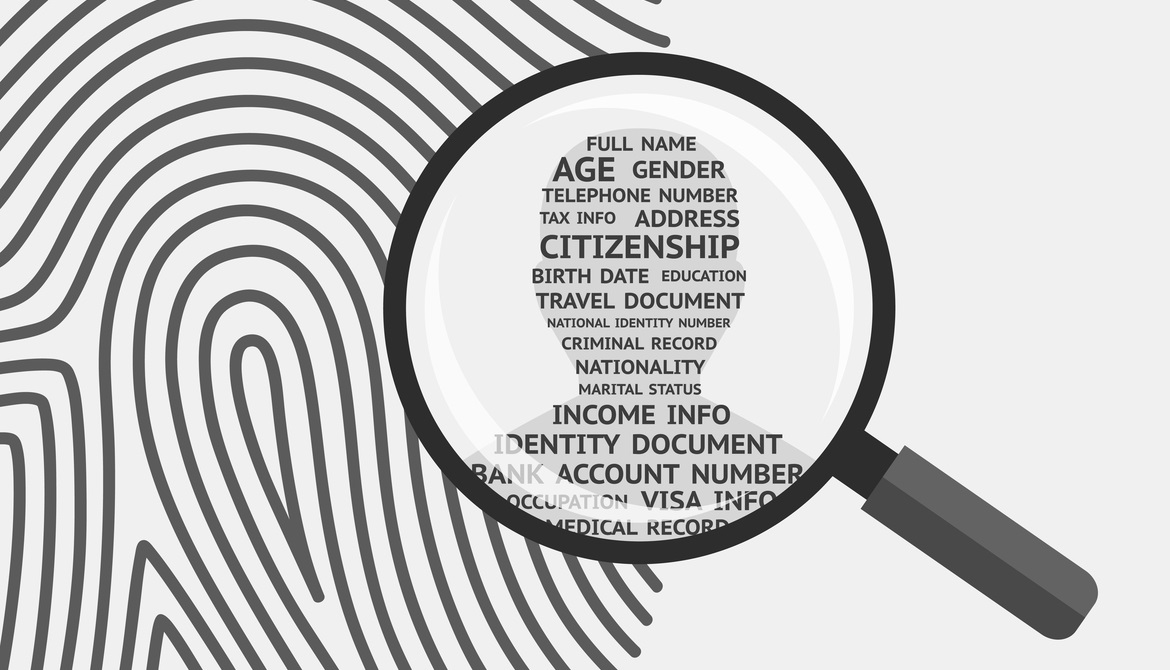
Privacy-preserving sensing in AIoT
Main contributors from the group: Linshan Jiang (topic coordinator), Chaojie Gu, Mengyao Zheng (alumnus), Dixing Xu (alumnus)
Privacy-Preserving Machine Learning in IoT
As explained in our post, a hybrid computing paradigm consisting of edge computing at the front end and cloud computing at the back end will prevail along with the formation of IoT as a global infrastructure. In addition, the deep neural network-based learning and inference will be important for improving the sensing performance of IoT systems. In a number of scenarios, the IoT edge and the cloud back end need to work together to implement AI-empowered sensing, during which privacy-sensitive data generated at the edge may be exchanged between the edge and the cloud. The group has ongoing research on designing and evaluating privacy-preserving learning and inference approaches in AIoT systems. Our challenge paper (PDF) provides a taxonomy of the existing privacy-preserving learning and inference approaches.